Process Instance Clustering based on Conformance Checking Artefacts
by Mathilde Boltenhagen
Thursday 21 October 2021 at 10h15
Room 2Z81 ENS Paris-Saclay as well as online
Zoom link: https://cnrs.zoom.us/j/95623460694
Passcode: FhrK69
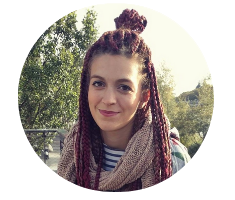
Abstract: As event data becomes an ubiquitous source of information, data science techniques represent an unprecedented opportunity to analyze and react to the processes that generate this data. Process Mining is an emerging field that bridges the gap between traditional data analysis techniques, like Data Mining, and Business Process Management. One core value of Process Mining is the discovery of formal process models like Petri nets or BPMN models which attempt to make sense of the events recorded in logs. Due to the complexity of event data, automated process discovery algorithms tend to create dense process models which are hard to interpret by humans. Fortunately, Conformance Checking, a sub-field of Process Mining, enables relating observed and modeled behavior, so that humans can map these two pieces of process information. Conformance checking is possible through alignment artefacts which associate process models and event logs. Different types of alignment artefacts exist, namely alignments, multi-alignments and anti-alignments. Currently, only alignment artefacts are deeply addressed in the literature. It allows to relate the process model to a given process instance. However, because many behaviors exist in logs, identifying an alignment per process instance hinders the readability of the log-to-model relationships.
The present thesis proposes to exploit the conformance checking artefacts for clustering the process executions recorded in event logs, thereby extracting a restrictive number of modeled representatives. Data clustering is a common method for extracting information from dense and complex data. By grouping objects by similarities into clusters, data clustering enables to mine simpler datasets which embrace the similarities and the differences contained in data. Using the conformance checking artefacts in a clustering approach allows to consider a reliable process model as a baseline for grouping the process instances. Hence, the discovered clusters are associated with modeled artefacts, that we call model-based trace variants, which provides opportune log-to-model explanations. From this motivation, we have elaborated a set of methods for computing conformance checking artefacts. The first contribution is the computation of a unique modeled behavior that represents of a set of process instances, namely multi-alignment. Then, we propose several alignment-based clustering approaches which provide clusters of process instances associated to a modeled artefact. Finally, we highlight the interest of anti-alignment for extracting deviations of process models with respect to the log. This latter artefact enables to estimate model precision, and we show its impact in model-based clustering. We provide SAT encoding for all the proposed techniques. Heuristic algorithms are then added to deal with computing capacity of today’s computers, at the expense of loosing optimality.
Jury:
- Marlon Dumas, University of Tartu, Reviewer
- Jochen De Weerdt, KU Leuven, Reviewer
- Pascal Poizat, Université Paris Nanterre, LIP6, Examiner
- Fatiha Zaïdi, Université Paris Saclay, Examiner
- Marco Montali, Free University of Bozen-Bolzano, Examiner
- Thomas Chatain, ENS Paris-Saclay, Advisor
- Josep Carmona, Universitat Politècnica de Catalunya, Advisor