Speaker: Pablo Barcelo, Chair of Computational Engineering, School of Engineering and Faculty of Mathematics, Universidad Católica de Chile
Tuesday 20th May 2025, 14:00, 1Z56 (to be confirmed)
Abstract:
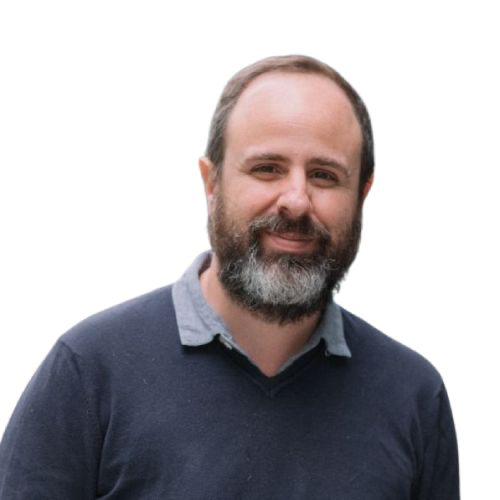
I present three case studies from my collaborative research that highlight the essential role of logic in enhancing our understanding of modern machine learning architectures. The first two examples focus on the expressive capabilities of two prominent architectures: Transformers, which have revolutionized NLP applications, and Graph Neural Networks, a leading approach for classifying graph-structured data. We employ temporal logic techniques to analyze the properties that Transformers can recognize, and modal logics to examine the properties discernible by Graph Neural Networks. The third example addresses the pursuit of explainable AI, demonstrating how first-order logic can be used to design languages that declare, evaluate, and compute explanations for decisions made by machine learning models.