https://calendar.google.com/calendar/ical/proval%40lri.fr/public/basic.ics
Lien iCal public :Seminars
Keynote: Challenges and triumphs of verification in the CSP style
Speaker: Bill Roscoe Emeritus professor, University of Oxford, GB
Thursday (!) May 23 2024, 14:00, Room 1Z56
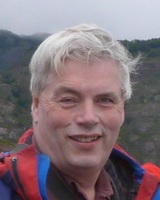
Abstract: I have been doing practical verification in CSP, its tools and models for 40 years. The main challenge has been packaging this for the industrial engineer. I will discuss how this has been solved in the Coco System www.cocotec.io, which is used for object based development of massive systems in industry. Separately I will show how I have used it to underpin a highly innovative blockchain consensus protocol by using it to model decentralised, partly malevolent systems.
Agenda
Séminaires antérieurs
Lindenmayer graph languages, first-order theories and expanders
Speaker: Teodor Knapik, Université de la Nouvelle-Calédonie, Nouméa
Tuesday 14 May 2024, 14:00, Room 1Z56
Abstract: Imagined by Kolmogorov in the middle of past century, expanders form remarkable graph families with applications in areas as diverse as robust communication networks and probabilistically checkable proofs, to name just two. Since the proof of the existence of expanders, it took several years to come up with an explicit algebraic construction [Margulis 1973] of some expander families. Their first elementary (combinatorial) construction has been published in 2002 and awarded Gödel Prize in 2009.
In this talk, we introduce a framework that captures most of the known combinatorial constructions of expanders. It is based on a generalisation of Lindenmayer systems to the domain of graphs. We call this formalism Lindenmayer graph grammars. We identify a few essential properties which make decidable the language checking problem with respect to first-order sentences. This result is obtained by encompassing a graph language into an automatic structure. By language checking in this specific context, we mean the following problem.
Instance: a Lindenmayer graph grammar and a first-order sentence. Question: Does there exist a graph in the language for which the sentence holds?
Static analysis and model reduction for a site-graph rewriting language
Speaker: Jérôme Feret ENS Ulm, jerome.feret@ens.psl.eu
Tuesday Mar 26 2024, 14:00, Room 1Z56
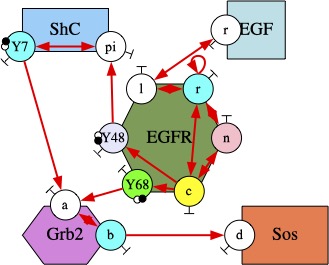
Abstract: Software sciences have a role to play in the description, the organization, the execution, and the analysis of the molecular interaction systems such as biological signaling pathways. These systems involve a huge diversity of bio-molecular entities whereas their dynamics may be driven by races for shared resources, interactions at different time- and concentration-scales, and non-linear feedback loops. Understanding how the behavior of the populations of proteins orchestrates itself from their individual interactions, which is the holy grail on systems biology, requires dedicated languages offering adapted levels of abstraction and efficient analysis tools.
In this talk we describe the design of formal tools for Kappa, a site-graph rewriting language inspired by bio-chemistry. In particular, we introduce a static analysis to compute some properties on the biological entities that may arise in models, so as to increase our confidence in them. We also present a model reduction approach based on a study of the flow of information between the different regions of the biological entities and the potential symmetries. This approach is applied both in the differential and in the stochastic semantics.
Atomic congestion games with non-separable costs: an application to smart charging
Speaker: Yezekaël Hayel, Université d'Avignon
Tuesday March 12 2024, 14:00, Room 1Z56
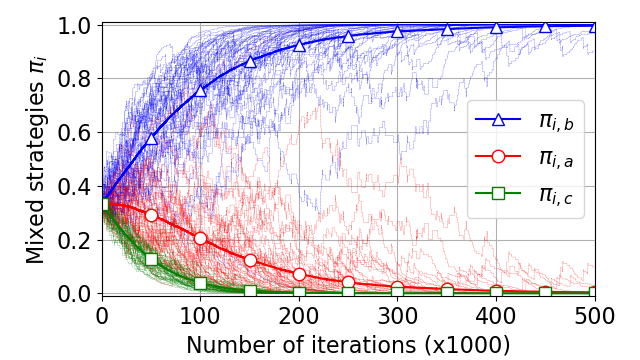
Abstract: Atomic congestion games with separable costs are a specific type of non-cooperative games with a finite number of players where the cost of a commodity depends on the number of players choosing it. But in many applications, resources may be correlated in the sense that the resource cost may depend on the usage of other resources, and thus cost function is non-separable. This is the case for traffic models with opposite directions dependencies, resource graph games, and smart charging games to cite a few examples. In this talk, after introducing the concepts of atomic congestion games with non-separable costs, a specific smart charging game will illustrate such game theoretical framework. In this particular setting, we prove the existence of pure Nash Equilibrium by showing ordinal potential function existence. We also demonstrate the convergence of a simple Reinforcement Learning algorithm to the pure NE for both synchronous and asynchronous versions. Finally, the recent framework of Resource Graph Games will be presented. In this setting, dependencies between resources are modeled as an oriented graph. This new framework generalizes atomic congestion games with non-separable costs and opens new questions about the existence and uniqueness of pure NE in this general setup.
Solving Quantified Boolean Formulas and its Applications
Speaker: Martina Seidel, Johannes Kepler University, Linz, Austria
Tuesday Feb 20 2024, 14:00, Room 1Z53
Abstract: Quantified Boolean Formulas (QBFs) extend propositional logic by quantifiers over the Boolean variables. Despite the PSPACE hardness of their decision problem, much progress has been made in practical solving, making QBFs an attractive framework for encoding various problems from artificial intelligence and formal verification.
In this talk, we will give an overview on recent trends and developments in QBF solving and we will discuss promising applications of QBFs.
Modèles de Langage
Speaker: Gustave Cortal, LMF
Tuesday Feb 06 2024, 14:00, 1Z71
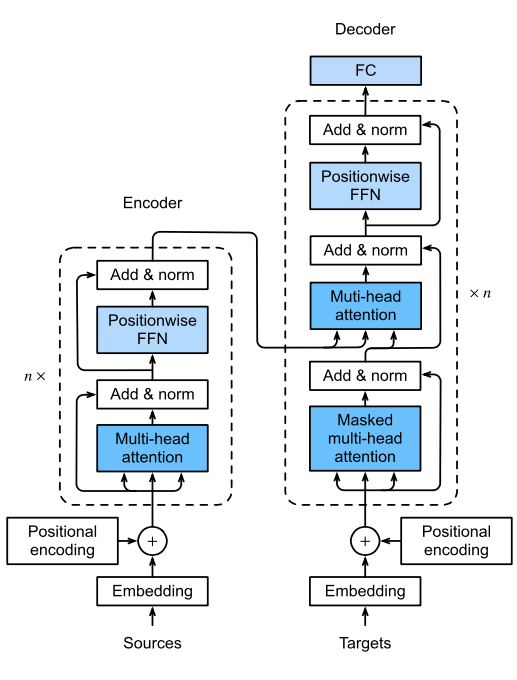
Abstract: Je propose d'introduire certains concepts clés du traitement automatique des langues. Le cours se concentre sur les modèles de langage, qui sont des modèles prédictifs calculant la probabilité d’une séquence de mots, et trouvant des applications en traduction, résumé de texte, agent conversationnel, etc.
Je parlerai de différentes architectures utilisées dans l’histoire pour la modélisation statistique du langage, comme les n-grammes, les réseaux de neurones feed-forward, les réseaux de neurones récurrents et les transformers. Les avantages et les inconvénients de chaque architecture seront exposés. À la fin, il sera possible de comprendre conceptuellement comment un modèle comme ChatGPT fonctionne.
Logics for Strategic Reasoning: Recent Developments and Application to Mechanism Design
Speaker: Munyque Mittelmann, University of Naples
Tuesday, 12 December 2023, 14:00, Room 1Z71
Abstract: In recent years a wealth of logic-based languages have been introduced to reason about the strategic abilities of autonomous agents in multi-agent systems. This talk presents some of these important logical formalisms, namely, the Alternating-time Temporal Logic and Strategy Logic. We discuss recent extensions of those formalisms to capture different degrees of satisfaction, as well as to handle uncertainty caused by the partial observability of agents and the intrinsic randomness of MAS. Finally, we describe the recent application of those formalisms for Mechanism Design and explain how they can be used either to automatically check that a given mechanism satisfies some desirable property, or to produce a mechanism that does it.
Markov Decision Processes with Sure Parity and Multiple Reachability Objectives
Speaker: Raphael Berthon, RWTH Aachen
Tuesday, 28 November 2023, 14:00, Room 1Z25
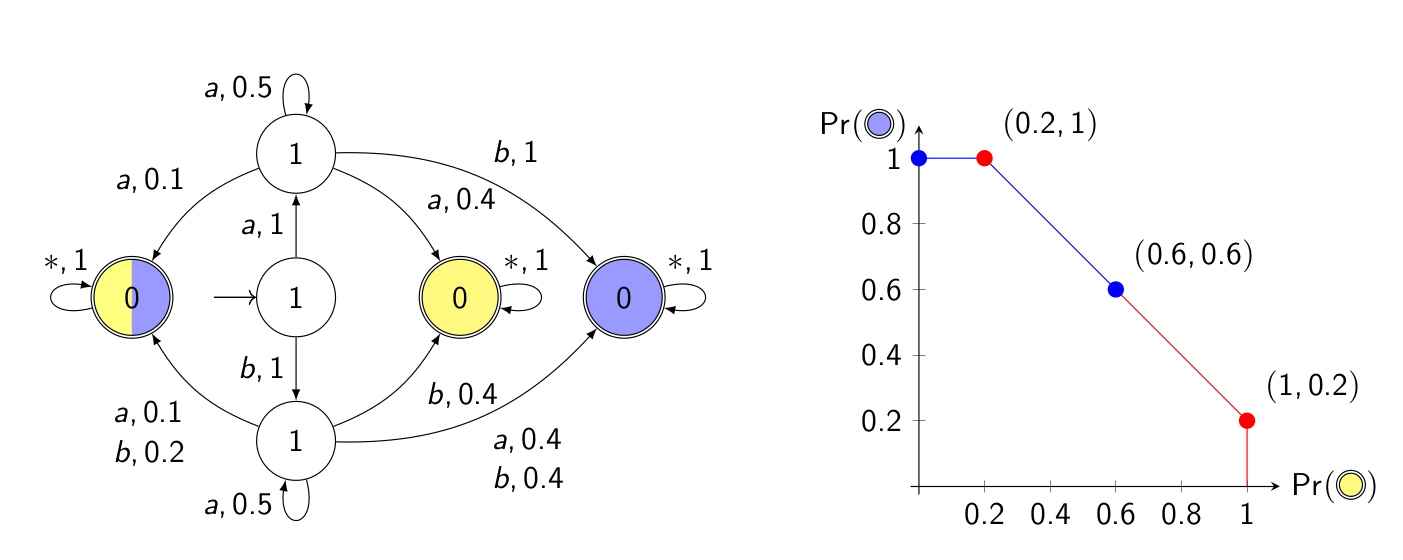
Abstract: When verifying multiple properties, it is sufficient to verify each individually, but when synthesizing strategies that satisfy multiple objectives, these objectives must be considered together. We consider the problem of finding strategies that satisfy a mixture of sure and threshold objectives in Markov decision processes. We focus on a single omega-regular objective expressed as parity that must be surely met while satisfying n reachability objectives with some probability thresholds too. We consider three combinations with a sure parity objective: (a) strict and (b) non-strict thresholds on all reachability objectives, and (c) maximizing the thresholds under a lexicographic ordering on the reachability objectives. We highlight the notion of projection for combining multiple objectives. We show that the decision variants of (a) are in PTIME, (b) in EXPTIME, and (c) can be solved by considering n parity games, and give associated algorithms.
Phase Semantics for Linear Logic with Least and Greatest Fixed Points
Speaker: Farzad Jafarrahmani, IRIT
Tuesday, 21 November 2023, 14:00, 1Z56
Abstract: The truth semantics of linear logic (i.e. phase semantics) is often overlooked despite having a wide range of applications and deep connections with several denotational semantics. In phase semantics, one is concerned about the provability of formulas rather than the contents of their proofs (or refutations). Linear logic equipped with the least and greatest fixpoint operators (\muMALL) has been an active field of research for the past one and a half decades. Various proof systems are known viz. finitary and non-wellfounded, based on explicit and implicit (co)induction respectively. In this talk, I present an extension of the phase semantics of multiplicative additive linear logic (a.k.a. \MALL) to \muMALL with explicit (co)induction (i.e. \muMALLind). Then I introduce a Tait-style system for \muMALL where proofs are wellfounded but potentially infinitely branching. We will see its phase semantics and the fact that it does not have the finite model property. This presentation is based on joint work with Abhishek De and Alexis Saurin.
Analysis of Parameterized Systems
Speaker: Chana Weil-Kennedy, Technical University Munich
Tuesday, 19 December 2023, 14:00, 1Z71
Abstract: My research project focuses on parameterized verification of distributed systems, where the parameter is often the number of agents present in the system. During my thesis with Javier Esparza in Munich, I studied this kind of problem for subclasses of Petri nets with application to population protocols, but also for systems communicating by broadcast or by shared register. Currently in my postdoc I am continuing the study of these systems with "simple" modes of communication, and I'm also starting to look at language inclusion problems. In the future, I want to continue to analyze distributed systems for which the parameterized problems are not immediately undecidable, by broadening the range of formal method techniques used.
Phase Semantics for Linear Logic with Least and Greatest Fixed Points
Speaker: Farzad Jafarrahmani, IRIT
Tuesday, 16 Janvier 2024, 14:00, 1Z76
Abstract: The truth semantics of linear logic (i.e. phase semantics) is often overlooked despite having a wide range of applications and deep connections with several denotational semantics. In phase semantics, one is concerned about the provability of formulas rather than the contents of their proofs (or refutations). Linear logic equipped with the least and greatest fixpoint operators (\muMALL) has been an active field of research for the past one and a half decades. Various proof systems are known viz. finitary and non-wellfounded, based on explicit and implicit (co)induction respectively. In this talk, I present an extension of the phase semantics of multiplicative additive linear logic (a.k.a. \MALL) to \muMALL with explicit (co)induction (i.e. \muMALLind). Then I introduce a Tait-style system for \muMALL where proofs are wellfounded but potentially infinitely branching. We will see its phase semantics and the fact that it does not have the finite model property. This presentation is based on joint work with Abhishek De and Alexis Saurin.